Model predicts scoliosis risk in patients receiving SMA treatment
Study finds algorithm identifies features in patients receiving DMTs
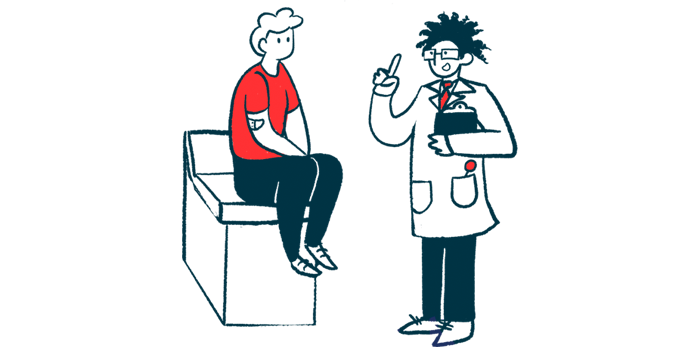
An algorithm that takes into account clinical measures of motor symptom severity alongside data on factors like age and height may help predict the risk of scoliosis in people with spinal muscular atrophy (SMA) who have received disease-modifying treatment.
“We trained a scoliosis classifier, demonstrating how data give rise to meaningful insights that can help physicians identify informative clinical features in a patient to aid diagnostics and improve scoliosis treatment and potentially downstream outcomes,” the researchers wrote.
Development of the algorithm was described in the study, “Feature Engineering for the Prediction of Scoliosis in 5q-Spinal Muscular Atrophy,” which was published in the Journal of Cachexia, Sarcopenia and Muscle.
SMA is a neuromuscular disorder that causes progressive muscle weakness and wasting. Weak trunk muscles can set the stage for scoliosis, an abnormal, sideways curve in the spine. Over the last decade, the landscape of the disease has shifted radically, and there are now three widely approved disease-modifying therapies that can stop or slow SMA progression: Evrysdi (risdiplam), Spinraza (nusinersen), and Zolgensma (onasemnogene abeparvovec-xioi).
The availability of multiple treatments to combat SMA progression has been revolutionary, and patients are achieving outcomes that have been unthinkable in the past. But this can put clinicians in a bit of a bind when it comes to predicting the risk of disease complications like scoliosis.
Risk factors in new SMA treatment era
Traditionally, scoliosis risk has been estimated largely based on the type of SMA that a person has. People with SMA type 1, who develop symptoms in early infancy, will almost always develop scoliosis in the long term, whereas scoliosis is less common in individuals with milder SMA type 2 and SMA type 3.
Here, researchers conducted a series of analyses aiming to identify factors that predict the risk of scoliosis in the modern era of disease-modifying therapies. The team collected data on 84 people with SMA, most with type 1, 2, or 3. Three patients did not have symptoms or their SMA type was unknown. Slightly more than half of the patients had scoliosis, and almost all had received one or more disease-modifying therapy.
The researchers analyzed the data using statistical models as well as machine learning. The latter involves feeding a computer data, along with mathematical rules (algorithms) that the computer uses to identify patterns in the data. The identified patterns can then be applied to make sense of new data.
The team ultimately zeroed in on several factors that showed consistent associations with scoliosis risk. Some of these were individual attributes like age, weight, and height.
“This corresponds with our understanding of scoliosis progression in SMA, where the natural history of SMA disease includes an increased lifetime risk of scoliosis,” the scientists wrote. “The risk of scoliosis is a function of the patient’s survival and stage of the disease.”
Measures of motor function, such as the Hammersmith Functional Motor Scale Expanded (HFMSE) and the six-minute walk test (6MWT) of exercise capacity, also showed significant associations with scoliosis risk. The risk of scoliosis also was higher in patients who used assistive devices to get around or had a history of using feeding tubes or ventilation.
The researchers noted that all of these factors “are easily assessed during patient visits and can help anticipate scoliosis onset.”
When the researchers put all these different factors together in machine learning models, they found they were able to predict the risk of scoliosis with an accuracy of about 82%. This was much more accurate than trying to predict scoliosis risk using traditional parameters like SMA type and age of onset.
“This new approach could allow us to provide earlier and better scoliosis treatment in a changing patient landscape,” the scientists wrote.