AI model designed to help predict scoliosis in SMA patients
Model could help doctors offer early intervention, personalized care for patients
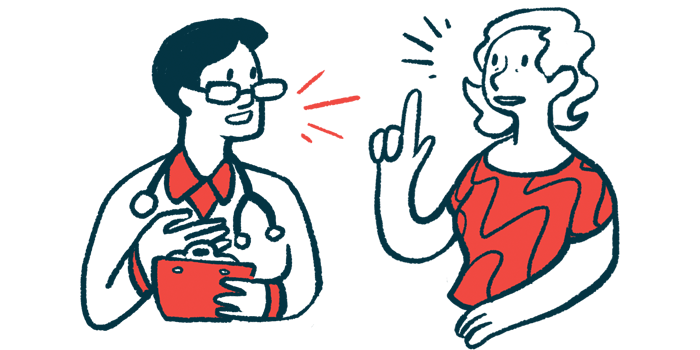
Researchers have developed an artificial intelligence (AI)-based algorithm to help predict the presence of scoliosis in spinal muscular atrophy (SMA) patients based on clinical features routinely collected at the doctor’s office.
“Our predictive model … could augment clinical decision-making in the future by offering quantified probabilities of scoliosis development, providing actionable insights that extend beyond the capabilities of traditional assessments,” researchers say.
“These insights would support targeted early intervention strategies and personalized care in neuromuscular disease management.”
The findings were published in a study, “An interpretable data-driven prediction model to anticipate scoliosis in spinal muscular atrophy in the era of (gene-) therapies,” in the journal Scientific Reports.
Most SMA patients develop scoliosis which can lead to breathing issues
Most SMA patients develop scoliosis, or an abnormal and progressive sideways curvature of the spine due to weakness in the back muscles that normally support it. This can ultimately give the lungs less room to expand, leading to breathing issues.
Proper management of scoliosis depends on being able to successfully identify it and monitor how quickly it is progressing. Historical data on how scoliosis usually progresses in SMA might not be as informative now that new SMA therapies, including gene therapy, can substantially change the disease course for patients.
The goal of the study was to develop an AI-based tool that could help clinicians predict whether an SMA patient has scoliosis based on easily collected clinical data. The algorithm learned to make predictions by analyzing clinical data from 86 SMA patients, some of whom had already been diagnosed with scoliosis via imaging studies.
Overall, six clinical features were incorporated into the predictive algorithm, including age, ventilation (breathing) support, use of orthoses — devices like braces or splints to help with problems in the limbs or spine — and contractures, where the muscles, tendons, or joints tighten and cause deformities. Also considered was performance on two standard tests of motor function in SMA.
AI model able to predict with 77% accuracy the presence of spinal deformity
These individual features alone didn’t have good predictive power for identifying scoliosis in the patients, but the AI model that took them into consideration collectively was 77% accurate at predicting the presence of the spinal deformity.
The model could also be used to map how a person’s scoliosis risk had changed over time, clearly showing an inflection point around the time of scoliosis onset for patients who had been diagnosed.
To validate the findings, the scientists applied their algorithm to a different set of 10 SMA patients it hadn’t been exposed to yet. Patients were then followed up clinically to verify whether the model’s prediction proved true. The model correctly predicted scoliosis probability for nine of these 10 patients.
Given their findings, the scientists believe the AI tool could be used in the clinic to “perform an automated scoliosis prediction based on routine feature inputs,” they wrote.
It could tell doctors which patients need a referral to a pediatric spine specialist, who in turn could use the data to help develop an individualized treatment plan.
AI model could make it easier for doctors to identify scoliosis
By making it easier for doctors to analyze who has scoliosis, the scientists also believe their model “has the potential to democratize expertise that is otherwise clustered at specialized centers.”
The researchers emphasized that the model is currently designed to predict the likelihood that a person has scoliosis at any given clinic visit. At this point, it “has not been trained to forecast the presence of scoliosis at a future visit,” the team wrote.
Additional training of the algorithm with larger datasets will help to make the model even better and more accurate, the researchers wrote.
“Future work entails giving access to and validating our model on a data set from an international clinic,” they concluded.